Researcher Explores Machine Learning’s Role in Predicting BRD Case Outcomes
K-State graduate student says this technology may lead to better cattle management decisions.
September 17, 2024
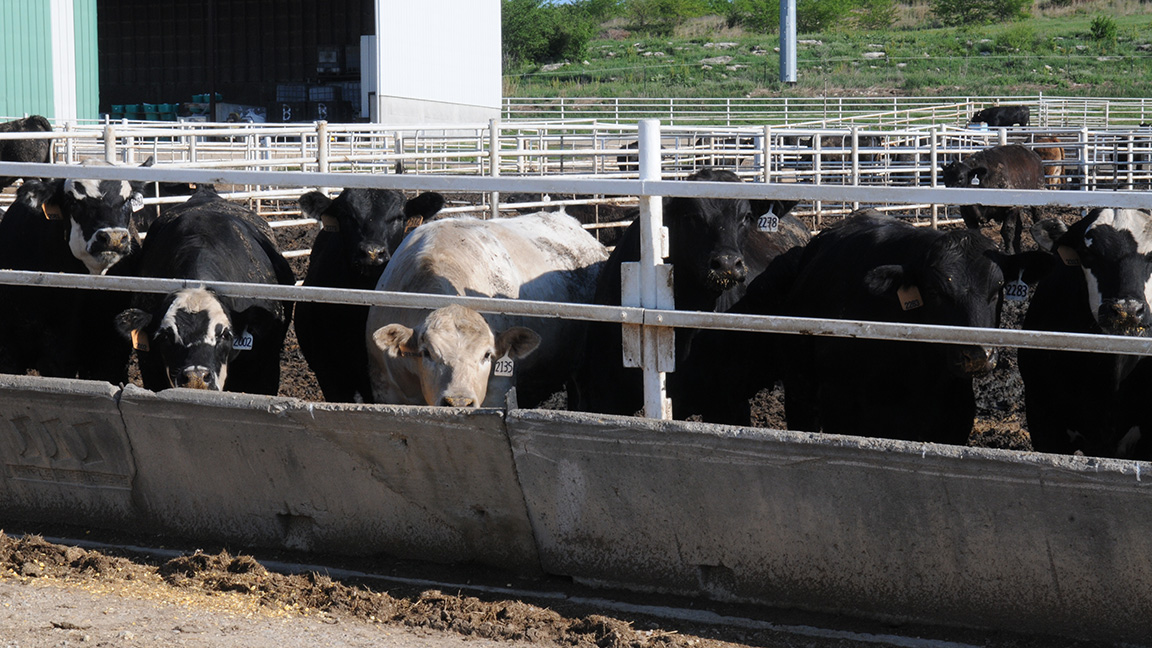
K-State graduate student Lilli Heinen says the key to increasing predictive model accuracy is continued interaction between computer scientists and beef production experts. [Photo provided by K-State.]
by Jacob Klaudt, K-State Research & Extension
Cattle feeders have dealt with bovine respiratory disease (BRD) for decades. Heightened through the stress brought on by transporting and commingling cattle, BRD has proven to be the costliest affliction within fed-cattle production.
Lilli Heinen, a Kansas State University (K-State) graduate student with the Beef Cattle Institute (BCI), says that with the cattle industry a long way from an outright cure and prevention method, predictive models crafted through machine learning may soon become a mainstream option for mitigating the effects of BRD.
Machine learning is a form of artificial intelligence in which machines — in this case, computers — learn and improve from data fed into the system.
“The main objective of my doctorate program is to get a better idea of how producers can use machine learning and predictive devices with feedlot datasets, which can be diverse and messy,” she says.
Other areas of agriculture — like crop production — have been using machine learning for things such as yield prediction for a while. Last year, Heinen and the BCI set out to learn whether the same concept applies to determining the outcomes for feedlot cattle treated for BRD.
“We used the program Microsoft Azure and data collected by feedyards at different processing times to develop five algorithms to discover BRD treatment results for individual cattle,” says Heinen.
“Our research evaluation compared all five of them against each other,” she says. “We took the true outcomes for those cattle, and then set those side by side with what the models said would happen, and then calculated the accuracy of each of them.”
Heinen said the technology shows promise, but requires improvement before real-world application.
“We found that these models performed with moderate accuracy,” she says. “Generally, more cattle live than die from BRD, so it forecasts the animals that will not die with higher exactness. We wanted to find ways to improve its ability to anticipate the animals that will perish.”
One factor that Heinen and researchers modified to improve precision includes creating models for individual feedyards.
“Originally, we had used one big dataset from multiple locations for one algorithm,” she says. “We eventually split that data up and made the models specific to each feedyard, which allowed them to make more accurate projections.”
When ready for use, predictive tools formed through machine learning may lessen the feedlot labor shortage while increasing production efficiency, according to Heinen.
“I am not saying that computers can replace a human decision; yet, we do not have the needed number of people in feedyards to make treatment decisions all the time.” — Lilli Heinen
“I am not saying that computers can replace a human decision; yet, we do not have the needed number of people in feedyards to make treatment decisions all the time,” she says. “Right now, many feedyards are struggling to keep cattle fed. If we could apply these instruments in a manner that would delegate decisions out of human hands, operators may use their time and labor resources elsewhere, where it might be more beneficial having a person make decisions.”
Heinen’s most current research regarding machine learning involves building models for groups of cattle — rather than individuals — to detect morbidity rate and limit antibiotic use.
“Depending on their morbidity rate (how many animals are sick within a group), these tools may help decide whether cattle should receive metaphylaxis, which is the administration of an antibiotic to every single animal in the group,” she said. “Hopefully, [machine learning tools] can help us lower the use of antibiotics and resistance to them.”
Editor’s note: Jacob Klaudt is a K-State Research & Extension news service writer.
Angus Beef Bulletin EXTRA, Vol. 16, No. 9-B
Topics: Equipment / Facilities , Feedyard , Health , News
Publication: Angus Beef Bulletin